Back in the 1990s, when US banks started installing automated teller machines in a big way, the human tellers who worked in those banks seemed to be facing rapid obsolescence. If machines could hand out cash and accept deposits on their own, around the clock, who needed people?
The banks did, actually. It’s true that the ATMs made it possible to operate branch banks with many fewer employees: 13 on average, down from 20. But the cost savings just encouraged the parent banks to open so many new branches that the total employment of tellers actually went up.
The robots are coming: SpaceX founder Elon Musk, and the late physicist Stephen Hawking both publicly warned that machines will eventually start programming themselves, and trigger the collapse of human civilization.
You can find similar stories in fields like finance, health care, education and law, says James Bessen, the Boston University economist who called his colleagues’ attention to the ATM story in 2015. “The argument isn’t that automation always increases jobs,” he says, “but that it can and often does.”
That’s a lesson worth remembering when listening to the increasingly fraught predictions about the future of work in the age of robots and artificial intelligence. Think driverless cars, or convincingly human speech synthesis, or creepily lifelike robots that can run, jump and open doors on their own: Given the breakneck pace of progress in such applications, how long will there be anything left for people to do?
In the early 1980s, automated teller machines began populating banks and stoking fears that the machines would make human bank tellers obsolete. But after an initial dip, the number of full-time bank workers actually began to rise.
That question has been given its most apocalyptic formulation by figures such as Tesla and SpaceX founder Elon Musk and the late physicist Stephen Hawking. Both have publicly warned that the machines will eventually exceed human capabilities, move beyond our control and perhaps even trigger the collapse of human civilization. But even less dramatic observers are worried. In 2014, when the Pew Research Center surveyed nearly 1,900 technology experts on the future of work, almost half were convinced that artificially intelligent machines would soon lead to accelerating job losses — nearly 50 percent by the early 2030s, according to one widely quoted analysis. The inevitable result, they feared, would be mass unemployment and a sharp upswing in today’s already worrisome levels of income inequality. And that could indeed lead to a breakdown in the social order.
“It’s always easier to imagine the jobs that exist today and might be destroyed than it is to imagine the jobs that don’t exist today and might be created.”
Jed KolkoOr maybe not. “It’s always easier to imagine the jobs that exist today and might be destroyed than it is to imagine the jobs that don’t exist today and might be created,” says Jed Kolko, chief economist at the online job-posting site Indeed. Many, if not most, experts in this field are cautiously optimistic about employment — if only because the ATM example and many others like it show how counterintuitive the impact of automation can be. Machine intelligence is still a very long way from matching the full range of human abilities, says Bessen. Even when you factor in the developments now coming through the pipeline, he says, “we have little reason in the next 10 or 20 years to worry about mass unemployment.”
So — which way will things go?
There’s no way to know for sure until the future gets here, says Kolko. But maybe, he adds, that’s not the right question: “The debate over the aggregate effect on job losses versus job gains blinds us to other issues that will matter regardless” — such as how jobs might change in the face of AI and robotics, and how society will manage that change. For example, will these new technologies be used as just another way to replace human workers and cut costs? Or will they be used to help workers, freeing them to exercise uniquely human abilities like problem-solving and creativity?
“There are many different possible ways we could configure the state of the world,” says Derik Pridmore, CEO of Osaro, a San Francisco-based firm that makes AI software for industrial robots, “and there are a lot of choices we have to make.”
Automation and jobs: lessons from the past
In the United States, at least, today’s debate over artificially intelligent machines and jobs can’t help but be colored by memories of the past four decades, when the total number of workers employed by US automakers, steel mills and other manufacturers began a long, slow decline from a high of 19.5 million in 1979 to about 17.3 million in 2000 — followed by a precipitous drop to a low of 11.5 million in the aftermath of the Great Recession of 2007–2009. (The total has since recovered slightly, to about 12.7 million; broadly similar changes were seen in other heavily automated countries such as Germany and Japan.) Coming on top of a stagnation in wage growth since about 1973, the experience was traumatic.
True, says Bessen, automation can’t possibly be the whole reason for the decline. “If you go back to the previous hundred years,” he says, “industry was automating at as fast or faster rates, and employment was growing robustly.” That’s how we got to millions of factory workers in the first place. Instead, economists blame the employment drop on a confluence of factors, among them globalization,the decline of labor unions, and a 1980s-era corporate culture in the United States that emphasized down-sizing, cost-cutting and quarterly profits above all else.
But automation was certainly one of those factors. “In the push to reduce costs, we collectively took the path of least resistance,” says Prasad Akella, a roboticist who is founder and CEO of Drishti, a start-up firm in Palo Alto, California, that uses AI to help workers improve their performance on the assembly line. “And that was, ‘Let’s offshore it to the cheapest center, so labor costs are low. And if we can’t offshore it, let’s automate it.’”
AI and robots in the workplace
Automation has taken many forms, including computer-controlled steel mills that can be operated by just a handful of employees, and industrial robots, mechanical arms that can be programmed to move a tool such as a paint sprayer or a welding torch through a sequence of motions. Such robots have been employed in steadily increasing numbers since the 1970s. There are currently about 2 million industrial robots in use globally, mostly in automotive and electronics assembly lines, each taking the place of one or more human workers.
The distinctions among automation, robotics and AI are admittedly rather fuzzy — and getting fuzzier, now that driverless cars and other advanced robots are using artificially intelligent software in their digital brains. But a rough rule of thumb is that robots carry out physical tasks that once required human intelligence, while AI software tries to carry out human-level cognitive tasks such as understanding language and recognizing images. Automation is an umbrella term that not only encompasses both, but also includes ordinary computers and non-intelligent machines.
AI’s job is toughest. Before about 2010, applications were limited by a paradox famously pointed out by the philosopher Michael Polanyi in 1966: “We can know more than we can tell” — meaning that most of the skills that get us through the day are practiced, unconscious and almost impossible to articulate. Polanyi called these skills tacit knowledge, as opposed to the explicit knowledge found in textbooks.
Imagine trying to explain exactly how you know that a particular pattern of pixels is a photograph of a puppy, or how you can safely negotiate a left-hand turn against oncoming traffic. (It sounds easy enough to say “wait for an opening in traffic” — until you try to define an “opening” well enough for a computer to recognize it, or to define precisely how big the gap must be to be safe.) This kind of tacit knowledge contained so many subtleties, special cases and things measured by “feel” that there seemed no way for programmers to extract it, much less encode it in a precisely defined algorithm.
Today, of course, even a smartphone app can recognize puppy photos (usually), and autonomous vehicles are making those left-hand turns routinely (if not always perfectly). What’s changed just within the past decade is that AI developers can now throw massive computer power at massive datasets — a process known as “‘deep learning.” This basically amounts to showing the machine a zillion photographs of puppies and a zillion photographs of not-puppies, then having the AI software adjust a zillion internal variables until it can identify the photos correctly.
Although this deep learning process isn’t particularly efficient — a human child only has to see one or two puppies — it’s had a transformative effect on AI applications such as autonomous vehicles, machine translation and anything requiring voice or image recognition. And that’s what’s freaking people out, says Jim Guszcza, US chief data scientist at Deloitte Consulting in Los Angeles: “Wow — things that before required tacit knowledge can now be done by computers!” Thus the new anxiety about massive job losses in fields like law and journalism that never had to worry about automation before. And thus the many predictions of rapid obsolescence for store clerks, security guards and fast-food workers, as well as for truck, taxi, limousine and delivery van drivers.
Meet my colleague, the robot
The fact is that, even now, it’s very hard to completely replace human workers.
But then, bank tellers were supposed to become obsolete, too. What happened instead, says Bessen, was that automation via ATMs not only expanded the market for tellers, but also changed the nature of the job: As tellers spent less time simply handling cash, they spent more time talking with customers about loans and other banking services. “And as the interpersonal skills have become more important,” says Bessen, “there has been a modest rise in the salaries of bank tellers,” as well as an increase in the number of full-time rather than part-time teller positions. “So it’s a much richer picture than people often imagine,” he says.
Similar stories can be found in many other industries. (Even in the era of online shopping and self-checkout, for example, the employment numbers for retail trade are going up smartly.) The fact is that, even now, it’s very hard to completely replace human workers.
Steel mills are an exception that proves the rule, says Bryan Jones, CEO of JR Automation, a firm in Holland, Michigan, that integrates various forms of hardware and software for industrial customers seeking to automate. “A steel mill is a really nasty, tough environment,” he says. But the process itself — smelting, casting, rolling, and so on — is essentially the same no matter what kind of steel you’re making. So the mills have been comparatively easy to automate, he says, which is why the steel industry has shed so many jobs.
A job is greater than its tasks: Every job, from janitor to CEO, is a mix of individual tasks that fall somewhere between hard to automate with today’s technology (red), and easy to automate (blue). At the same time, each type of task makes up a certain percentage (circle size) of the work in any given industry sector. Taken together, these measures suggest that a sector such as manufacturing (second row from top) may be ripe for additional automation because it still involves quite a lot of predictable physical work (large blue circle, right). In contrast, the healthcare and social assistance industry (fifth row from bottom), requires managing others and using expertise (red circles, left), tasks that aren’t very feasible for automated systems.
When people are better
“Where it becomes more difficult to automate is when you have a lot of variability and customization,” says Jones. “That’s one of the things we’re seeing in the auto industry right now: Most people want something that’s tailored to them,” with a personalized choice of color, accessories or even front and rear grills. Every vehicle coming down the assembly line might be a bit different.
It’s not impossible to automate that sort of flexibility, says Jones. Pick a task, and there’s probably a laboratory robot somewhere that has mastered it. But that’s not the same as doing it cost-effectively, at scale. In the real world, as Akella points out, most industrial robots are still big, blind machines that go through their motions no matter who or what is in the way, and have to be caged off from people for safety’s sake. With machines like that, he says, “flexibility requires a ton of retooling and a ton of programming — and that doesn't happen overnight.”
Contrast that with human workers, says Akella. The reprogramming is easy: “You just walk onto the factory floor and say, ‘Guys, today we’re making this instead of that.’” And better still, people come equipped with abilities that few robot arms can match, including fine motor control, hand-eye coordination and a talent for dealing with the unexpected.
All of which is why most automakers today don’t try to automate everything on the assembly line. (A few of them did try it early on, says Bessen. But their facilities generally ended up like General Motors’ Detroit-Hamtramck assembly plant,which quickly became a debugging nightmare after it opened in 1985: Its robots were painting each other as often as they painted the Cadillacs.) Instead, companies like Toyota, Mercedes-Benz and General Motors restrict the big, dumb, fenced-off robots to tasks that are dirty, dangerous and repetitive, such as welding and spray-painting. And they post their human workers to places like the final assembly area, where they can put the last pieces together while checking for alignment, fit, finish and quality — and whether the final product agrees with the customer’s customization request.
To help those human workers, moreover, many manufacturers (and not just automakers) are investing heavily in collaborative robots, or “cobots” — one of the fastest-growing categories of industrial automation today.
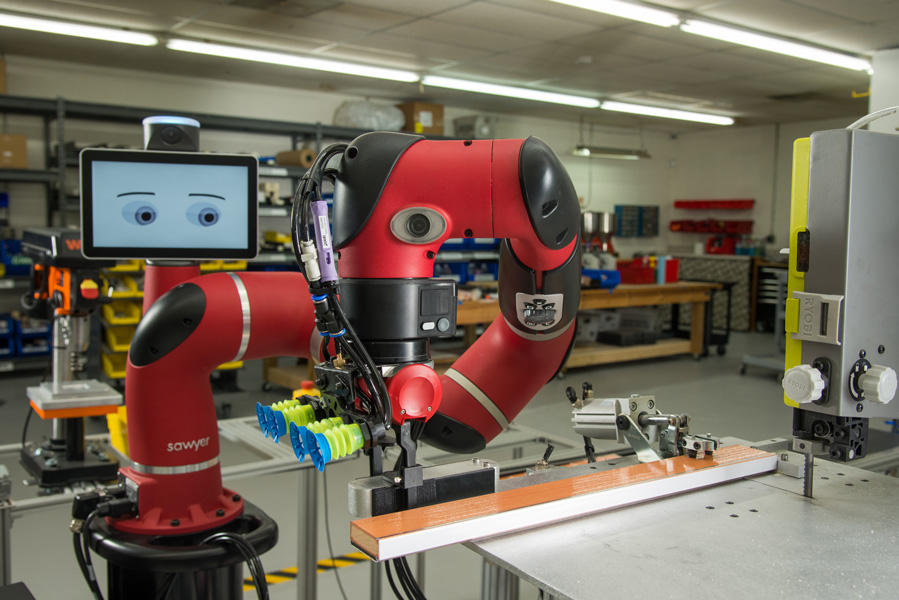
Sawyer, a collaborative robot made by Rethink Robotics, is one of many such “cobots” designed to work safely alongside humans on the shop floor. Sawyer guides its movements with a computer vision system, uses force feedback to know how hard it is gripping (and to keep from crushing things), and can be trained to do a new task simply by guiding its 7-jointed arm through the required motion. The expression of the eyes on the display screen change to indicate Sawyer’s status, from “working well” to “needs attention.”
CREDIT: COURTESY OF RETHINK ROBOTICS INC.
Collaborative robots: Machines work with people
Cobots are now available from at least half a dozen firms. But they are all based on concepts developed by a team working under Akella in the mid-1990s, when he was a staff engineer at General Motors. The goal was to build robots that are safe to be around, and that can help with stressful or repetitive tasks while still leaving control with the human workers.
To get a feel for the problem, says Akella, imagine picking up a battery from a conveyor belt, walking two steps, dropping it into the car and then going back for the next one — once per minute, eight hours per day. “I've done the job myself,” says Akella, “and I can assure you that I came home extremely sore.” Or imagine picking up a 150-pound “cockpit” — the car’s dashboard, with all the attached instruments, displays and air-conditioning equipment — and maneuvering it into place through the car’s doorway without breaking anything.
Devising a robot that could help with such tasks was quite a novel research challenge at the time, says Michael Peshkin, a mechanical engineer at Northwestern University in Evanston, Illinois, and one of several outside investigators that Akella included in his team. “The field was all about increasing the robots’ autonomy, sensing and capacity to deal with variability,” he says. But until this project came along, no one had focused too much on the robots’ ability to work with people.
So for their first cobot, he and his Northwestern colleague Edward Colgate started with a very simple concept: a small cart equipped with set of lifters that would hoist, say, the cockpit, while the human worker guided it into place. But the cart wasn’t just passive, says Peshkin: It would sense its position and turn its wheels to stay inside a “virtual constraint surface” — in effect, an invisible midair funnel that would guide the cockpit through the door and into position without a scratch. The worker could then check the final fit and attachments without strain.

Cobots can be adapted to help human workers in a wide variety of manufacturing environments. At MS Schramberg, a mid-sized magnet manufacturer in Baden-Württemberg, Germany, multiple collaborative robots called Sawyers have been deployed to relieve workers from some of the most repetitive assembly tasks.
CREDIT: COURTESY OF RETHINK ROBOTICS INC.
Another GM-sponsored prototype replaced the cart with a worker-guided robotic arm that could lift auto components while hanging from a movable suspension point on the ceiling. But it shared the same principle of machine assistance plus worker control — a principle that proved to be critically important when Peshkin and his colleagues tried out their prototypes on General Motors’ assembly line workers.
“We expected a lot of resistance,” says Peshkin. “But in fact, they were welcoming and helpful. They totally understood the idea of saving their backs from injury.” And just as important, the workers loved using the cobots. They liked being able to move a little faster or a little slower if they felt like it. “With a car coming along every 52 seconds,” says Peshkin, “that little bit of autonomy was really important.” And they liked being part of the process. “People want their skills to be on display,” he says. “They enjoy using their bodies, taking pleasure in their own motion.” And the cobots gave them that, he says: “You could swoop along the virtual surface, guide the cockpit in and enjoy the movement in a way that fixed machinery didn’t allow.”
AI and its limits
Akella’s current firm, Drishti, reports a similarly welcoming response to its AI-based software. Details are proprietary, says Akella. But the basic idea is to use advanced computer vision technology to function somewhat like a GPS for the assembly line, giving workers turn-by-turn instructions and warnings as they go. Say that a worker is putting together an iPhone, he explains, and the camera watching from overhead believes that only three out of four screws were secured: “We alert the worker and say, ‘Hey, just make sure to tighten that screw as well before it goes down the line.’”
This does have its Big Brother aspects, admits Drishti’s marketing director, David Prager. “But we’ve got a lot of examples of operators on the floor who become very engaged and ultimately very appreciative,” he says. “They know very well the specter of automation and robotics bearing down on them, and they see very quickly that this is a tool that helps them be more efficient, more precise and ultimately more valuable to the company. So the company is more willing to invest in its people, as opposed to getting them out of the equation.”
This theme — using technology to help people do their jobs rather than replacing people — is likely to be a characteristic of AI applications for a long time to come. Just as with robotics, there are still some important things that AI can’t do.
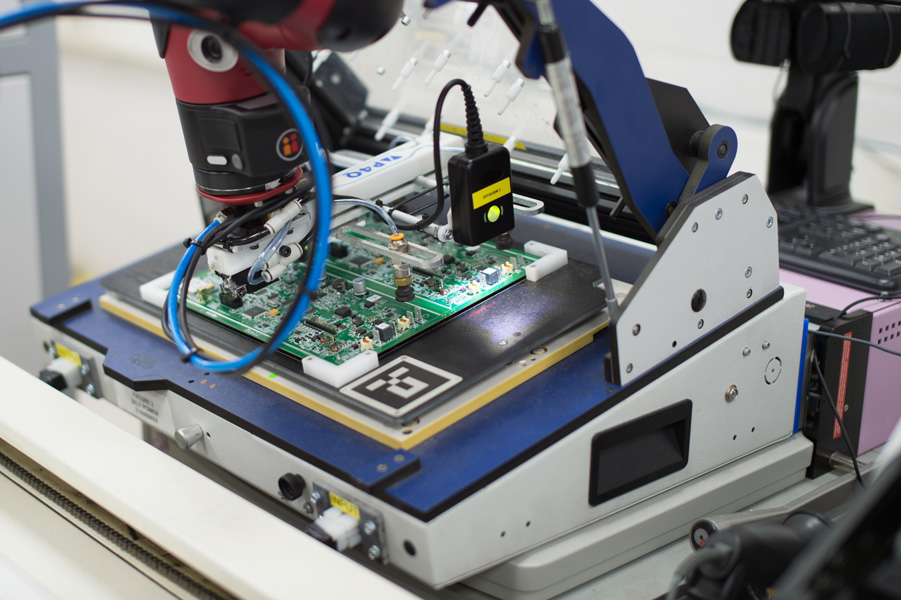
Robot arms can be equipped with “hands,” or grippers, that are specialized for the specific job. Here, Sawyer is using a gripper consisting of an array of suction cups to position a circuit board very precisely in a testing stand.
CREDIT: COURTESY OF RETHINK ROBOTICS INC.
Take medicine, for example. Deep learning has already produced software that can interpret X rays as well as or better than human radiologists, says Darrell West, a political scientist who studies innovation at the Brookings Institution in Washington, DC. “But we’re not going to want the software to tell somebody, ‘You just got a possible cancer diagnosis,’” he says. “You're still going to need a radiologist to check on the AI, to make sure that what it observed actually is the case” — and then, if the results are bad, a cancer specialist to break the news to the patient and start planning out a course of treatment.
Likewise in law, where AI can be a huge help in finding precedents that might be relevant to a case — but not in interpreting them, or using them to build a case in court. More generally, says Guszcza, deep-learning-based AI is very good at identifying features and focusing attention where it needs to be. But it falls short when it comes to things like dealing with surprises, integrating many diverse sources of knowledge and applying common sense — “all the things that humans are very good at.”
And don’t ask the software to actually understand what it’s dealing with, says Guszcza. During the 2016 election campaign, to test Google’s Translate utility, he tried a classic experiment: Take a headline — “Hillary slams the door on Bernie” — then ask Google to translate it from English to Bengali and back again. Result: “Barney slam the door on Clinton.” A year later, after Google had done a massive upgrade of Translate using deep learning, Guszcza repeated the experiment with the result: “Hillary Barry opened the door.”
“I don’t see any evidence that we’re going to achieve full common-sense reasoning with current AI,” he says, echoing a point made by many AI researchers themselves. In September 2017, for example, deep learning pioneer Geoffrey Hinton, a computer scientist at the University of Toronto, told the news site Axios that the field needs some fundamentally new ideas if researchers ever hope to achieve human-level AI.
Job evolution
AI’s limitations are another reason why economists like Bessen don’t see it causing mass unemployment anytime soon. “Automation is almost always about automating a task, not the entire job,” he says, echoing a point made by many others. And while every job has at least a few routine tasks that could benefit from AI, there are very few jobs that are all routine. In fact, says Bessen, when he systematically looked at all the jobs listed in the 1950 census, “there was only one occupation that you could say was clearly automated out of existence — elevator operators.” There were 50,000 in 1950, and effectively none today.
On the other hand, you don’t need mass unemployment to have massive upheaval in the workplace, says Lee Rainie, director of internet and technology research at the Pew Research Center in Washington, DC. “The experts are hardly close to a consensus on whether robotics and artificial intelligence will result in more jobs, or fewer jobs,” he says, “but they will certainly change jobs. Everybody expects that this great sorting out of skills and functions will continue for as far as the eye can see.”
Worse, says Rainie, “the most worried experts in our sample say that we’ve never in history faced this level of change this rapidly.” It’s not just information technology, or artificial intelligence, or robotics, he says. It’s also nanotechnology, biotechnology, 3-D printing, communication technologies — on and on. “The changes are happening on so many fronts that they threaten to overwhelm our capacity to adjust,” he says.
Preparing for the future of work
If so, the resulting era of constant job churn could force some radical changes in the wider society. Suggestions from Pew’s experts and others include an increased emphasis on continuing education and retraining for adults seeking new skills, and a social safety net that has been revamped to help people move from job to job and place to place. There is even emerging support in the tech sector for some kind of guaranteed annual income, on the theory that advances in AI and robotics will eventually transcend the current limitations and make massive workplace disruptions inevitable, meaning that people will need a cushion.
This is the kind of discussion that gets really political really fast. And at the moment, says Rainie, Pew’s opinion surveys show that it’s not really on the public’s radar: “There are a lot of average folks, average workers saying, ‘Yeah, everybody else is going to get messed up by this — but I’m not. My business is in good shape. I can’t imagine how a machine or a piece of software could replace me.’”
But it’s a discussion that urgently needs to happen, says West. Just looking at what’s already in the pipeline, he says, “the full force of the technology revolution is going to take place between 2020 and 2050. So if we make changes now and gradually phase things in over the next 20 years, it’s perfectly manageable. But if we wait until 2040, it will probably be impossible to handle.”
Editor’s note: This story was updated on August 1 to correct the details of an experiment by Jim Guszcza. The story originally said that an experiment during the 2016 election campaign was conducted to see how much deep learning had improved Google’s Translate ability; in fact, the 2016 experiment was conducted before Google had fully upgraded Translate with deep learning. The initial test was done with the headline “Hillary slams the door on Bernie,” not “Bernie slams the door on Hillary” as originally stated. The headline that resulted after translation from English to Bengali and back again was "Barney slam the door on Clinton," not “Barry is blaming the door at the door of Hillary's door.” The deep-learning improvements were tested a year later with the same initial headline and the resulting headline after the translation to Bengali and back was “Hillary Barry opened the door.”