On a November evening in 1895, German physicist Wilhelm Röntgen was running an electrical current through a glass tube filled with gas to learn more about how such tubes emitted light. The scientist had covered the tube with black carARard, but to his surprise — though the lab was in darkness — he saw a light-reactive screen nearby fluorescing brightly.
Röntgen soon found that the mysterious invisible rays coming from the tube could penetrate his body, such that he could see flesh glowing around his bones on this screen. He replaced the screen with photographic film — and captured the world’s first X-ray image, revealing that the inner workings of the body could be made visible without surgery.
Röntgen took an X-ray of his wife Anna’s hand, complete with wedding ring. She apparently did not care for it (“I have seen my death,” she said), but the revolution in medical imaging that X-rays triggered has meant life for countless others.
Now a new frontier is opening up that promises to help this field save even more lives: Artificial intelligence (AI) is helping to analyze medical images. AI systems known as deep neural networks promise to help doctors sift through the huge amounts of at-times incomprehensible data gathered from imaging technologies to make lifesaving diagnoses, such as cancerous spots in X-rays.
“Image recognition is a solved problem for computers,” says biomedical informatician Andrew Beam of Harvard Medical School. “That’s a task deep learning will do better than the average doctor — full stop.”
Artificial brains
In an artificial neural network, software models of neurons are fed data and cooperate to solve a problem, such as recognizing abnormalities in X-rays. The neural net repeatedly adjusts the behavior of its neurons and sees if these new patterns of behavior are better at solving the problem. Over time, the network discovers which patterns are best at computing solutions. It then adopts these as defaults, mimicking the process of learning in the human brain.
A renaissance in artificial intelligence has taken place in the past decade with the advent of so-called deep neural networks. Whereas typical neural networks arrange their neurons in a few layers, each focused on handling one aspect of a problem, a deep neural network can have many layers, often more than a thousand. This, in turn, enhances its capabilities to analyze increasingly complex problems.
These systems became practical with the aid of graphical processing units (GPUs), the kind of microchips used to render images in video game consoles, because they can process visual data at high speeds. In addition, databases holding vast amounts of medical images with which to train deep neural networks are now commonplace.
Deep neural networks made their splash in 2012 when one known as AlexNet won an overwhelming victory in the best-known worldwide computer vision competition, ImageNet Classification. This work rapidly accelerated research and development in the field of “deep learning,” and the subject now dominates major conferences, including ones on medical imaging. The hope is that deep neural nets can help physicians deal with the flood of information they now must contend with.
The first half-hour of this University of California TV video provides a good primer on deep neural nets and their application in one, particular scenario: identifying from X-rays a condition called pneumothorax, in which air builds up in the chest cavity and collapses the lungs.
CREDIT: UNIVERSITY OF CALIFORNIA TELEVISION (UCTV) VIA YOUTUBE
A deluge of data
Nearly 400 million medical imaging procedures are now performed annually in the United States, according to the American Society of Radiologic Technologists. X-rays, ultrasounds, MRI scans and other medical imaging techniques are by far the largest and fastest-growing source of data in health care — researchers at IBM, which developed Watson and other deep-learning AIs used in commercial applications such as weather forecasting and tax preparation, estimate that they represent at least 90 percent of all current medical data.
But analyses of medical images still require human interpretation, leaving them vulnerable to human error. Properly identifying diseases such as cancer from medical images is tedious work that can be challenging even for knowledgeable specialists, as the abnormalities in an image that hint at such diseases can prove difficult to spot or confusing to diagnose. A 2015 study in the Journal of the American Medical Association, for example, found the chances of two pathologists analyzing tissue samples from breasts and agreeing on whether or not they had signs of atypia — a benign lesion of the breast that indicates an increased risk of breast cancer — were only 48 percent.
Moreover, the sheer number of medical images now generated can prove overwhelming to even the best experts. The IBM researchers estimated that in some hospital emergency rooms, radiologists have to deal with as many as 100,000 medical images daily.
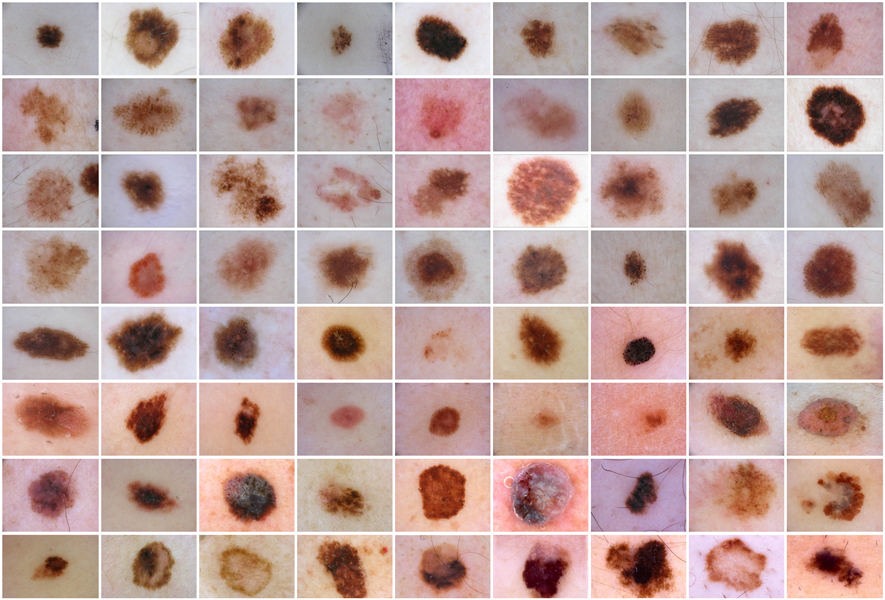
This grid shows an array of skin lesions, some benign and some malignant. A deep neural net for skin cancer detection would be trained on thousands of images so that the algorithm could “learn” to recognize the features of probable cancers. Some of the patterns it homes in on may be undetectable by human beings.
CREDIT: INTERNATIONAL SKIN IMAGING COLLABORATION
Errors in medical imaging analysis can take their toll on human lives. A key example is breast cancer, which the National Cancer Institute estimates will kill nearly 41,000 women in 2018 in the United States. Breast cancer screening involves analyzing mammograms, or low-energy X-rays of breasts, to identify suspicious abnormalities. If breast cancer is found early enough, it can often be cured with a nearly 100 percent survival rate, according to the American Cancer Society.
However, doctors can miss about 15 percent to 35 percent of breast cancers in screened women, because they either cannot see the cancers or misinterpret what they do see. In addition to these false negatives, mammography can yield false positives in 3 percent to 12 percent of cases — often because lesions appear suspicious on mammograms and look abnormal in needle biopsies. Patients undergo painful and expensive surgeries to remove them, but 90 percent turn out to be benign.
One can hear similar stories with other diseases. “You see someone come into your office from a rural area where they don’t have an expert physician around, and they have very late melanomas, and you think, ‘If they had come to my office earlier, I could have saved a life,’ ” says dermatologist Holger Haenssle at the University of Heidelberg, Germany. “Every skin cancer may be a person’s fate. If you detect a melanoma very early, you get complete healing with no side effects. So we’re struggling to become better.”
The skin is prone to a plethora of diseases, only some of which are malignant. This schematic tree displays the main classes; there are more than 2,000 in total.
Strategies to improve breast-cancer screening have included more frequent screening, regularly getting second opinions on mammograms, and new imaging technologies to make potential cancers more visible. Now, artificial neural networks promise to make medical imaging for breast cancer, and in general, smarter and more efficient.
The power of deep neural nets
In May, Haenssle and his colleagues found their deep neural network performed better than experienced dermatologists at detecting skin cancer. They first trained the neural net by showing it more than 100,000 images, including ones of malignant melanomas, the most lethal form of skin cancer, and benign moles. They told the net the diagnosis for each image.
The researchers then tested their neural net and 58 dermatologists from around the world against detailed skin images. Whereas the dermatologists accurately diagnosed 88.9 percent of malignant melanomas and 75.7 percent of lesions that were not cancer, the neural net accurately diagnosed 95 percent of malignant melanomas and 82.5 percent of benign moles.
“We had 30 global experts who thought, ‘Nothing can beat me,’ but the computer was better,” Haenssle says. “The machine was superior to the physicians, even the most experienced ones.”
In an experiment, a deep neural network was tested to see how well it could identify melanoma, the most dangerous type of skin cancer. The algorithm — known as a convolutional neural network — was trained with more than 100,000 images of benign skin lesions and skin tumors then tested against 58 dermatologists on 100 images that included 20% melanomas. This graph plots the “true positive” rate (how well the scorer identified actual melanomas) against “false positives” (harmless lesions misidentified as melanomas). A good scorer would have a high true positive rate and a low false positive rate, and score toward the top-left corner of the graph. Dermatologists scored well, as shown by the green dot representing an average for all 58. (Red, blue and orange dots are expert, skilled and beginner groups, respectively.) But the neural network did better, as shown by the teal line with diamonds, each of which uses a different probability cut-off for scoring a lesion as malignant. Physicians performing on the same level as the algorithm will be positioned on, or very close to, the curve. Physicians performing worse will appear below the curve; physicians performing better will appear above or left of the curve.
These findings suggest that neural nets could prove to be lifesaving. Skin cancer is the most common cancer in the United States, according to the Centers for Disease Control and Prevention, and early detection via neural nets could have a major impact on whether one survives it. While the five-year survival rate for melanoma is approximately 15 percent to 20 percent if it is detected in its latest stages, it rises to about 97 percent if discovered in its earliest states, according to the American Cancer Society.
Similar promising findings have occurred with breast cancer, cervical cancer, lung cancer, heart failure, diabetic retinopathy, potentially dangerous lung nodules and prostate cancer, among other diseases.
Adding the human touch
Although deep neural nets are currently not in clinical use for medical imaging, a few are in clinical trials (Beam expects many more trials before long). For example, biomedical engineer Anant Madabhushi at Case Western Reserve University in Cleveland and his colleagues are applying a deep neural net to analyze digitized biopsy samples at Tata Memorial Hospital in Mumbai, India. The aim is to predict the outcomes of early-stage breast cancer to see which women require chemotherapy and which don’t, providing low-cost diagnoses in parts of the world that cannot afford more expensive conventional approaches, Madabhushi says.
But even though neural nets can outperform humans on image recognition, that doesn’t mean doctors are out of a job. For one thing, Beam notes, while machines are currently good at perceptual tasks such as seeing and hearing, they are nowhere near as good at long chains of reasoning — skills needed for deciding which treatment is best for a given patient, or for a specific population of patients. “We shouldn’t over-interpret the successes we’ve had so far,” he says. “A general-purpose medical AI is still a long way off.”
And while scientists may train a neural net to spot a specific anomaly better than a person could, this net might not do as well if one sought to train it to recognize many different kinds of anomalies. “You can play chess against a computer, and it will win, but it you try to get a computer to play all the board games in the world, it will not be as good with its results,” Haenssle says.
The future of deep neural nets will likely have them working in conjunction with physicians instead of replacing them. For example, in 2016 scientists at Harvard developed a deep neural net that could distinguish cancer cells from normal breast-tissue cells with 92.5 percent accuracy. In this case, pathologists beat the computers with a 96.6 percent accuracy, but when the deep neural net’s predictions were combined with a pathologist’s diagnoses, the resulting accuracy was 99.5 percent.
The many advances made with X-rays stem in part from Röntgen’s deliberate decision to not patent his discovery so that the world could benefit freely from his work. More than a century after Röntgen won the first Nobel Prize in physics in 1901, artificial brains promise to advance medical imaging to breakthroughs he might not have ever imagined. “We are excited about the future,” Haenssle says.